The Limitations of Relying Solely on ChatGPT in Machine Learning
Written on
Chapter 1: The Bigger Picture of Machine Learning
The recent spotlight on an intelligent chatbot capable of engaging in conversations on a wide array of topics might suggest a groundbreaking development. However, as someone who has closely followed the evolution of machine learning tools over the years, I feel compelled to emphasize that numerous companies are actively developing user-friendly software that leverages advanced automation, presenting significant competitive benefits.
Contrary to popular belief, integrating machine learning into a business does not necessitate an influx of costly data scientists creating intricate programming solutions that could disrupt the entire organization. Instead, it begins with leveraging straightforward data structures that managers are already accustomed to and utilizing low-code or no-code tools to build menu-driven algorithms. This enables the formation of models, their education, testing their predictive power, refining them, and implementing them with relative ease.
Section 1.1: Real-World Applications of Machine Learning
My experience as a strategic advisor for BigML over the past decade has afforded me the opportunity to observe countless projects undertaken by our clients globally. BigML has emerged as a robust and profitable entity, securing eight patents in 2022 alone. Its tools are frequently utilized in educational settings by thousands of universities and boast a substantial portfolio of loyal customers worldwide. While I believe BigML stands out among competitors, it is merely one of many companies propelling the corporate embrace of machine learning technologies.
Subsection 1.1.1: Diverse Use Cases
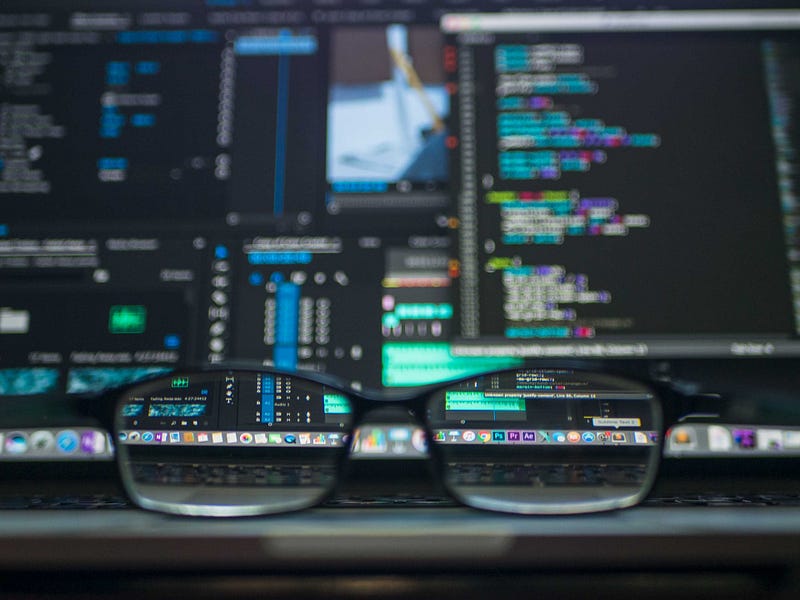
The applications of BigML’s tools are extensive, spanning industries such as banking—where they assist in credit risk assessment and fraud detection—highway management, railroad infrastructure, demand forecasting, retail promotions, contract negotiation, and research in specialized fields like echocardiography. Additionally, they enhance call center operations and optimize demand generation in B2C enterprises, among many other business scenarios.
These examples represent only a fraction of the projects clients have consented to share. Many other initiatives exist, often discussed only in industry gatherings or machine learning conferences, yet they hold far greater conceptual significance and added value compared to a chat interface, regardless of its perceived intelligence.
Section 1.2: The Limitations of Large Language Models
While OpenAI's ChatGPT has indeed raised awareness about the potential of machine learning, we must not confine our understanding and adoption to large language models alone. Although these models may significantly influence the future of search engines and other fields, they are not necessarily the most promising aspect of machine learning.
Chapter 2: Embracing Broader Machine Learning Opportunities
We can hope that the introduction of ChatGPT, as the first widely accessible machine learning application, will foster greater acceptance of this transformative technology and its myriad implications. However, it is crucial to recognize that there is a vast landscape of machine learning possibilities beyond conversational tools, even if they lack verbal communication.
The first video titled "Should we let students use ChatGPT? | Natasha Berg | TEDxSioux Falls" discusses the implications of ChatGPT in educational settings and raises critical questions about its role in learning environments.
In the second video, "Do you think that ChatGPT can reason?" the discussion revolves around the reasoning capabilities of ChatGPT and its potential impact on various fields, encouraging viewers to consider the broader implications of AI.