Harnessing AI to Revolutionize Waste Management and Recycling
Written on
Chapter 1: The Importance of a Circular Economy
Aiming for a circular economy is crucial for reducing carbon emissions. In this model, discarded products and materials are repurposed effectively, extending their lifecycle. Annually, the production and incineration of plastic alone contribute approximately 400 million tons of CO2—equivalent to the emissions from 183,000 nonstop flights for a year! Alarmingly, only a third of produced plastic is recycled, while metals face even lower recycling rates. Enhancing recycling processes could significantly mitigate these emissions.
To optimize recycling, the European Union mandates that member states categorize waste into four primary categories: paper, plastic, glass, and organic matter. Since this sorting task is highly repetitive, automation is a viable solution. However, effective automation hinges on the ability to identify discarded items and their materials, allowing robots to sort waste efficiently. This is where artificial intelligence (AI) plays a pivotal role, simplifying and enhancing recycling efforts.
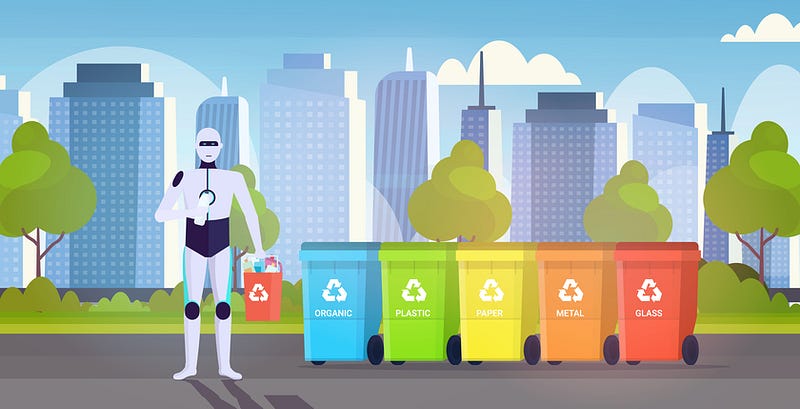
Chapter 2: Training AI for Effective Waste Sorting
Before AI can be deployed for waste separation, it must undergo rigorous training to identify various items and materials. This training process comprises several key steps:
- Data Collection: Initially, a vast number of images of diverse waste types are captured. Most of these images assist the neural network in recognizing distinct object characteristics.
- Categorization: These images are then manually classified into material types—such as paper, plastic, glass, and organic—enabling the neural network to learn effectively. Neural networks mimic the human brain's structure, functioning as a form of artificial intelligence.
- Data Augmentation: To ensure the intelligent scanner can recognize various iterations of the same waste item, transformations like rotation are applied to the images. Skipping this step could hinder the neural network's performance.
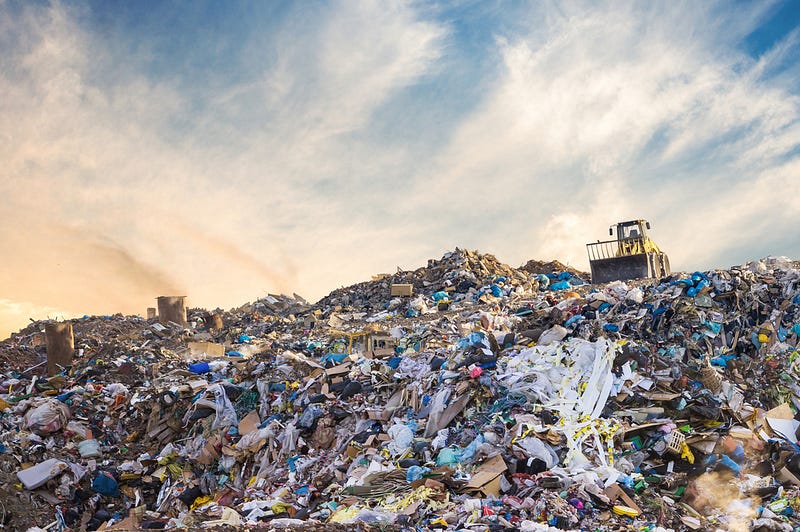
- Feature Detection: The neural network analyzes fundamental image properties, such as lines, edges, textures, and shadows. This step is complex, as computers interpret images as pixels rather than objects, necessitating advanced recognition capabilities.
- Information Simplification: The complexity of images must be reduced to retain only the most relevant information.
- Data Storage: The relevant information is compiled into a list of features and stored within the neural network.
Utilizing pre-trained neural networks can expedite this process, as these networks have already been trained on millions of images, refining their understanding of image characteristics.
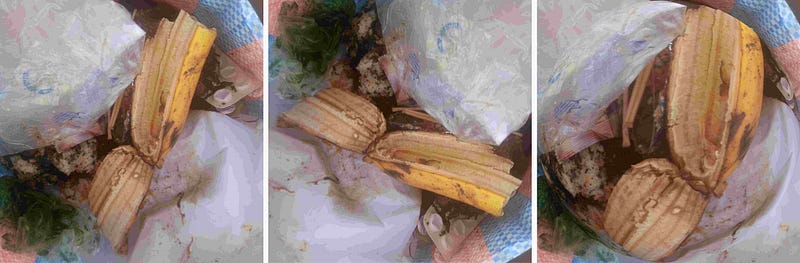
Chapter 3: Validating AI Recognition Capabilities
After the training phase, the remaining images are allocated for validation. This step is essential to ensure that the validation process employs images the neural network has not previously encountered, guaranteeing that the outcomes are genuinely attributed to the AI's capabilities.
With this training and validation methodology, over 85% of materials—including paper, plastic, glass, and organic waste—can be accurately identified, outperforming human recognition. However, certain materials remain challenging to differentiate, particularly plastic and glass. As neural networks continuously learn, their stored characteristics evolve, enhancing their ability to recognize waste items, including bottles.
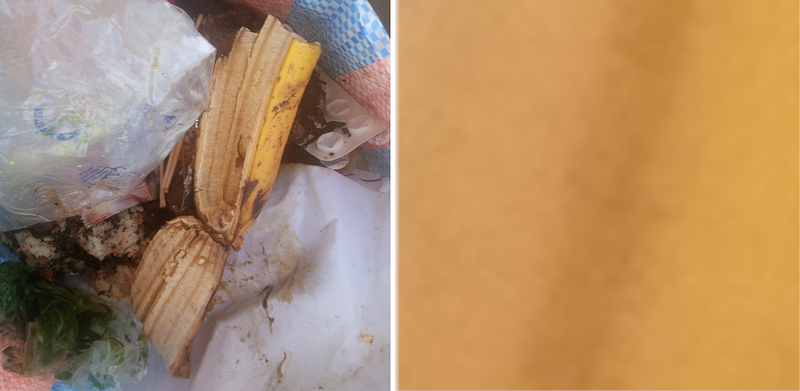
Conclusion
In summary, artificial intelligence significantly simplifies the recycling process by enabling the automated identification of various objects and materials. For this automation to function effectively, thorough training of the neural network is essential. Once trained, AI can identify new waste items and improve its recognition capabilities over time.
This video explores how artificial intelligence aids recycling facilities in identifying and sorting waste.
This video showcases tech startups leveraging AI and robotics to enhance recycling efficiency.